Classification of Pneumonia, Tuberculosis, and COVID-19 on Computed Tomography Images using Deep Learning
DOI:
https://doi.org/10.48048/tis.2023.6974Keywords:
Deep learning, Computed tomography image, Pneumonia, Tuberculosis, COVID-19Abstract
The accurate diagnosis of pneumonia, tuberculosis, and COVID-19 using computed tomography (CT) images is critical for radiologists. Artificial intelligence (AI) has been introduced as a tool to aid in rapid diagnosis. In this study, we evaluated 4 deep learning models, including AlexNet, GoogleNet, ResNet, and deep convolutional neural network (DCNN), to classify CT images of tuberculosis, pneumonia, and COVID-19. We collected 2,134 normal images, 943 images of tuberculosis, 2,041 images of pneumonia, and 3,917 images of COVID-19 from online datasets. To assess the efficiency of the models, we measured their image classification performance such as accuracy, F1 score, and area under the curve. Our performance evaluation indicated that ResNet was the highest-performing model, with the best accuracy, F1 score, and area under the curve (0.966, 0.931, 0.954, respectively). The second-best performing model was DCNN, while AlexNet and GoogleNet had the next-best performance, respectively. The deep learning models exhibit a capability that could be viewed as a substitute for predicting lung diseases and could be employed to support radiologists in CT image screening.
HIGHLIGHTS
- The four deep learning models including AlexNet, GoogleNet, ResNet, and DCNN can classified for 4 classes (tuberculosis, pneumonia, COVID-19, and normal)
- The AUC indicates the model's ability to distinguish between positive and negative images, ResNet had the highest AUC for normal, pneumonia, and COVID-19, while AlexNet was highest for tuberculosis
- The ResNet model performed the best in terms of accuracy of model prediction, correctly predicting the labels for all 4 classes of input data (tuberculosis, pneumonia, COVID-19, and normal)
GRAPHICAL ABSTRACT
Downloads
Metrics
References
E Bercovich and CM Javitt. Medical imaging: From roentgen to the digital revolution, and beyond. Rambam Maimonides Med. J. 2018; 9, e0034.
J Hsieh and T Flohr. Computed tomography recent history and future perspectives. J. Med. Imag. 2021; 8, 052109.
World Health Organization. Weekly epidemiological update on COVID-19 - 20 April 2022, Available at: https://www.who.int/publications/m/item/weekly-epidemiological-update-on-COVID-19--20-april-2022, accessed April 2022.
S Machnicki, D Patel, A Singh, A Talwar, B Mina, M Oks, P Makkar, D Naidich, A Mehta, NS Hill, KK Brown and S Raoof. The usefulness of chest CT imaging in patients with suspected or diagnosed COVID -19 a review of literature. Chest 2021; 160, 652-70.
S Du, S Gao, G Huang, S Li, W Chong, Z Jia, G Hou, YXJ Wáng and L Zhang. Chest lesion CT radiological features and quantitative analysis in RT-PCR turned negative and clinical symptoms resolved COVID-19 patients. Quant. Imag. Med. Surg. 2020; 10, 1307-17.
AS Bhalla, A Goyal, R Guleria and AK Gupta. Chest tuberculosis: Radiological review and imaging recommendations. Indian J. Radiol. Imag. 2015; 25, 213-25.
C Beigelman-Aubry and S Schmidt. Pulmonary infections: Imaging with CT. In: U Schoepf and F Meinel (Eds.). Multidetector-row CT of the thorax. Springer, Cham, Switzerland, 2016.
R Eibel and J Mueller. Pulmonary infections: Pneumonia. MRI Lung 2017; 8, 383-400.
M Carotti, F Salaffi, P Sarzi-Puttini, A Agostini, A Borgheresi, D Minorati, M Galli, D Marotto and A Giovagnoni. Chest CT features of coronavirus disease 2019 (COVID-19) pneumonia: Key points for radiologists. Radiol. Med. 2020; 125, 636-46.
MM Hefeda. CT chest findings in patients infected with COVID-19: Review of literature. Egypt. J. Radiol. Nucl. Med. 2020; 51, 239.
X Wang, C Liu, L Hong, C Yuan, J Ding, Q Jia, G Sun, W Peng and Q Sun. CT findings of patients infected with SARS-CoV-2. BMC Med. Imag. 2020; 20, 70.
AH Hoog, HK Meme, HV Deutekom, AM Mithika, C Olunga, F Onyino and MW Borgdorff. High sensitivity of chest radiograph reading by clinical officers in a tuberculosis prevalence survey. Int. J. Tuberc. Lung Dis. 2011; 15, 1308-14.
J Melendez, CI Sánchez, RH Philipsen, P Maduskar, R Dawson, G Theron, K Dheda and BV Ginneken. An automated tuberculosis screening strategy combining X-ray-based computer-aided detection and clinical information. Sci. Rep. 2016; 29, 25265.
N Sourlos, J Wang, Y Nagaraj, PV Ooijen and R Vliegenthart. Possible bias in supervised deep learning algorithms for CT lung nodule detection and classification. Cancers 2022; 14, 3867.
L Alzubaidi, J Zhang, AJ Humaidi, A Al-Dujaili, Y Duan, OJ Santamaría, MA Fadhel, M Al-Amidie and L Farhan. Review of deep learning: Concepts, CNN architectures, challenges, applications, future directions. J. Big Data 2021; 8, 53.
S Wang, G Cao, Y Wang, S Liao, Q Wang, J Shi, C Li and D Shen. Review and prospective: artificial intelligence in advanced medical imaging. Front. Radiol. 2021; 1, 781862.
R Ahmad. The role of digital technology and artificial intelligence in diagnosing medical images: A systematic review. Open J. Radiol. 2021; 11, 19-34.
T Wang, Y Lei, Y Fu, FJ Wynne, JW Curran, T Liu and X Yang. A review on medical imaging synthesis using deep learning and its clinical applications. J Appl. Clin. Med. Phys. 2020; 22, 11-36.
T Mahmood, J Li, Y Pei, F Akhtar, MU Rehman and SH Wasti. Breast lesions classifications of mammographic images using a deep convolutional neural network-based approach. PLoS One 2022; 17, e0263126.
D Castillo, V Lakshminarayanan and JM Rodríguez-Álvarez. MR images, brain lesions, and deep learning. Appl. Sci. 2021, 11, 1675.
R Li, C Xiao, Y Huang, H Hassan and B Huang. Deep learning applications in computed tomography images for pulmonary nodule detection and diagnosis: A review. Diagnostics 2022; 12, 298.
AM Heuvelmans, MAP van Ooijen, S Ather, FC Silva, D Han, CP Heussel, W Hickes, HU Kauczor, P Novotny, H Peschl, M Rook, R Rubtsov, OV Stackelberg, MT Tsakok, C Arteta, J Declerck, T Kadir, L Pickup, F Gleeson and M Oudkerk. Lung cancer prediction by deep learning to identify benign lung nodules. Lung Cancer 2021; 154, 1-4.
M Aminu, AN Ahmad and MHM Noor. Covid-19 detection via deep neural network and occlusion sensitivity maps. Alex. Eng. J. 2021; 60, 4829-55.
MD Ibrahim, MN Elshennawy and MA Sarhan. Deep-chest: Multi-classification deep learning model for diagnosing COVID-19, pneumonia, and lung cancer chest diseases. Comput. Biol. Med. 2021; 132, 104348.
TKS Kieu, A Bade, MHA Hijazi and H Kolivand. A survey of deep learning for lung disease detection on medical images: State-of-the-Art, taxonomy, issues and future directions. J. Imag. 2020; 6, 131.
G Du, X Cao, J Liang, X Chen and Y Zhan. Medical image segmentation based on U-Net: A review. J. Imag. Sci. Tech. 2020; 64, art00009.
Dive into deep learning, Available at: https://d2l.ai/chapter_recurrent-modern/encoder-decoder.html, accessed January 2022.
V Badrinarayanan, A Kendall and R Cipolla. SegNet: A deep convolutional encoder-decoder architecture for image segmentation. IEEE Trans. Pattern Anal. Mach. Intell. 2017; 39, 2481-95.
O Ronneberger, P Fischer and T Brox. U-Net convolutional networks for biomedical image segmentation. In: N Navab, J Hornegger, W Wells and A Frangi (Eds.). Medical image computing and computer-assisted intervention - MICCAI 2015. Springer, Cham, Switzerland.
X Xia and B Kulis. W-Net: A deep model for fully unsupervised image segmentation, Available https://doi.org/10.48550/arXiv.1711.08506, accessed January 2022.
E Mique Jr and A Malicdem. Deep residual U-Net based lung image segmentation for lung disease detection. IOP Conf. Ser. Mater. Sci. Eng. 2020; 803, 012004.
BA Skourt, AE Hassani and A Majda. Lung CT image segmentation using deep neural networks, Procedia Comput. Sci. 2018; 127, 109-13.
A Saood and I Hatem. COVID-19 lung CT image segmentation using deep learning methods: U-Net versus SegNet. BMC Med. Imaging. 2021; 21,19.
J Park, H Yang, HJ Roh, W Jung and GJ Jang. Encoder-weighted W-Net for unsupervised segmentation of cervix region in colposcopy images. Cancers 2022; 14, 3400.
W Zhao, H Chen and Y Lu. W-net: A network structure for automatic segmentation of organs at risk in thorax computed tomography. In: Proceedings of the 2020 2nd International Conference on Intelligent Medicine and Image Processing, Tianjin, China. 2020, p. 66-9.
H Alshazly, C Linse, M Abdalla, E Barth and T Martinetz. Covid-nets. Deep CNN architectures for detecting COVID-19 using chest CT scans. PeerJ Comput. Sci. 2021; 7, e655.
D Yang, C Martinez, L Visuña, H Khandhar, C Bhatt and J Carretero. Detection and analysis of COVID‑19 in medical images using deep learning techniques. Sci. Rep. 2021; 11, 19638.
MD Ibrahim, MN Elshennawy and MA Sarhan. Deep-chest: Multi-classification deep learning model for diagnosing COVID-19, pneumonia, and lung cancer chest diseases. Comput. Biol. Med. 2021; 132, 104348.
P Lakhani and B Sundaram. Deep learning at chest radiography: Automated classification of pulmonary tuberculosis by using convolutional neural networks. Radiology 2017; 284, 574-82.
W Tan, P Liu, X Li, Y Liu, Q Zhou, C Chen, Z Gong, X Yin and Y Zhang. Classification of COVID-19 pneumonia from chest CT images based on reconstructed super-resolution images and VGG neural network. Health Inf. Sci. Syst. 2021; 9, 10.
Y Song, S Zheng, L Li, X Zhang, XD Zhang, Z Huang, J Chen, R Wang, H Zhao, Y Chong, J Shen, Y Zha, Y Yang. Deep learning enables accurate diagnosis of novel coronavirus (COVID-19) with CT images. IEEE ACM Trans. Comput. Biol. Bioinformatics 2021; 18, 2775-80.
X Xu, X Jiang, C Ma, P Du, X Li, S Lv, L Yu, Y Chen, J Su, G Lang, Y Li, Hong Zhao, K Xu, L Ruan and W Wu. Deep learning system to screen coronavirus disease 2019 Pneumonia, Available at: https://doi.org/10.48550/arXiv.2002.09334, accessed January 2022.
R Nair, A Alhudhaf, D Koundal, R I Doewes and P Sharma. Deep learning-based COVID-19 detection system using pulmonary CT scans. Turk. J. Elec. Eng. Comput. Sci. 2021; 29, 2716-27.
SH Wang, DR Nayak, DS Guttery, X Zhang and YD Zhang. COVID-19 classification by CCSHNet with deep fusion using transfer learning and discriminant correlation analysis. Inform. Fusion 2021; 68, 131-48.
Kaggle dataset, Available at: https://www.kaggle.com/datasets, accessed January 2022.
Github, Available at: https://www.github.com, accessed January 2022.
Radiopaedia, Available at: https://radiopaedia.org, accessed January 2022.
Mendeley dataset, Available at: https://data.mendeley.com/, accessed January 2022.
Colaboratory, Available at: https://colab.research.google.com/, accessed February 2022.
CT Lung Segmentation, Available at: https://github.com/kairess/CT_lung_segmentation, accessed January 2022.
F Idrees, M Rajarajan, M Conti, TM Chen and Y Rahulamathavan. PIndroid: A novel Android malware detection system using ensemble learning methods. Comput. Secur. 2017; 68, 36-46.
P Ranganathan and R Aggarwal. Understanding the properties of diagnostic tests - Part 2: Likelihood ratios. Perspect. Clin. Res. 2018; 9, 99-102.
CM Florkowski. Sensitivity, specificity, receiver-operating characteristic (ROC) curves and likelihood ratios: Communicating the performance of diagnostic tests. Clin. Biochem. Rev. 2008; 29, S83-S87.
C Zheng, X Deng, Q Fu, Q Zhou, J Feng, H Ma, W Liu and C Zheng. A weakly-supervised framework for COVID-19 classification and lesion localization from Chest CT. IEEE Trans. Med. Imag. 2020; 39, 2615-25.
L Li, L Qin, Z Xu, Y Yin, X Wang, B Kong, J Bai, Y Lu, Z Fang, Q Song, K Cao, D Liu, G Wang, Q Xu, X Fang, S Zhang, J Xia and J Xia. Using artificial intelligence to detect COVID-19 and community-acquired pneumonia based on pulmonary CT: Evaluation of the diagnostic accuracy. Radiology 2020; 296, E65-E71.
S Walvekar and S Shinde. Detection of COVID-19 from CT images using resnet50. In: Proceedings of the 2nd International Conference on Communication & Information Processing, Kuala Lumpur Malaysia. 2020, p. 1-8.
I Lahsaini, MEH Daho and MA Chikh. Deep transfer learning-based classification model for covid-19 using chest CT-scans. Pattern Recognit. Lett. 2021; 152, 122-8.
XQ Zhang, GY Wang and SG Zhao. CapsNet-COVID19: Lung CT image classification method based on CapsNet model. Math. Biosci. Eng. 2022; 19, 5055-74.
R Wang, T Lei, R Cui, B Zhang and H Meng. Medical image segmentation using deep learning: A survey. IET Image Process. 2022; 16, 1243-67.
J Moorthy and UD Gandhi. A Survey on medical image segmentation based on deep learning techniques. Big Data Cogn. Comput. 2022; 6, 117.
XX Yin, L Sun, Y Fu, R Lu and Y Zhang. U-Net-based medical image segmentation. J. Healthc Eng. 2022; 2022, 4189781.
N Siddique, S Paheding, CP Elkin and V Devabhaktuni. U-Net and its variants for medical image segmentation: A review of theory and applications. IEEE Access. 2021; 9, 82031-57.
S Minaee, Y Boykov, F Porikli, A Plaza, N Kehtarnavaz and D Terzopoulos. Image segmentation using deep learning: A survey, Available at: https://doi.org/10.48550/arXiv.2001.05566, accessed January 2022.
O Rukundo. Effects of image size on deep learning. Electronics 2023; 12, 985.
CF Sabottke and BM spieler. The effect of image resolution on deep learning in radiology. Radiol. Artif. Intell. 2020; 2, e190015.
V Thambawita, I Strümke, SA Hicks, P Halvorsen, S Parasa and MA Riegler. Impact of image resolution on deep learning performance in endoscopy image classification: An experimental study using a large dataset of endoscopic images. Diagnostics 2021; 11, 2183.
X Ying. An overview of overfitting and its solutions. J. Phys. Conf. Ser. 2019; 1168, 022022.
C Shorten and TM Khoshgoftaar. A survey on image data augmentation for deep learning. J. Big Data 2019; 6, 60.
K Purohit, A Kesarwani, DR Kisku and M Dalui. COVID-19 detection on chest X-ray and CT scan images using multi-image augmented deep learning model. bioRxiv 2020, https://doi.org/10.1101/2020.07.15.205567
STH Kieu, A Bade, MHA Hijazi and H Kolivand. A survey of deep learning for lung disease detection on medical images: state-of-the-art, taxonomy, issues and future directions. J. Imag. 2020; 6, 131.
S Masoudi, SA Harmon, S Mehralivand, SM Walker, H Raviprakash, U Bagci, PL Choyke and B Turkbey. Quick guide on radiology image pre-processing for deep learning applications in prostate cancer research. J. Med. Imag. 2021; 8, 010901.
G Philip and S George. A review of preprocessing methods and pre-trained models for COVID-19 detection using medical images. Int. J. Eng. Res. Tech. 2022; 10, 236-9.
A Giełczyk, A Marciniak, M Tarczewska and Z Lutowski. Pre-processing methods in chest X-ray image classification. PLoS ONE 2022; 17, e0265949.
FA Dawood and ZM Abood. The importance of contrast enhancement in medical images analysis and diagnosis. Int. J. Eng. Res. Tech. 2018; 7, 21-4.
MW Mirza, A Siddiq and IR Khan. A comparative study of medical image enhancement algorithms and quality assessment metrics on COVID-19 CT images. Signal Image Video Process. 2023; 17, 915-24.
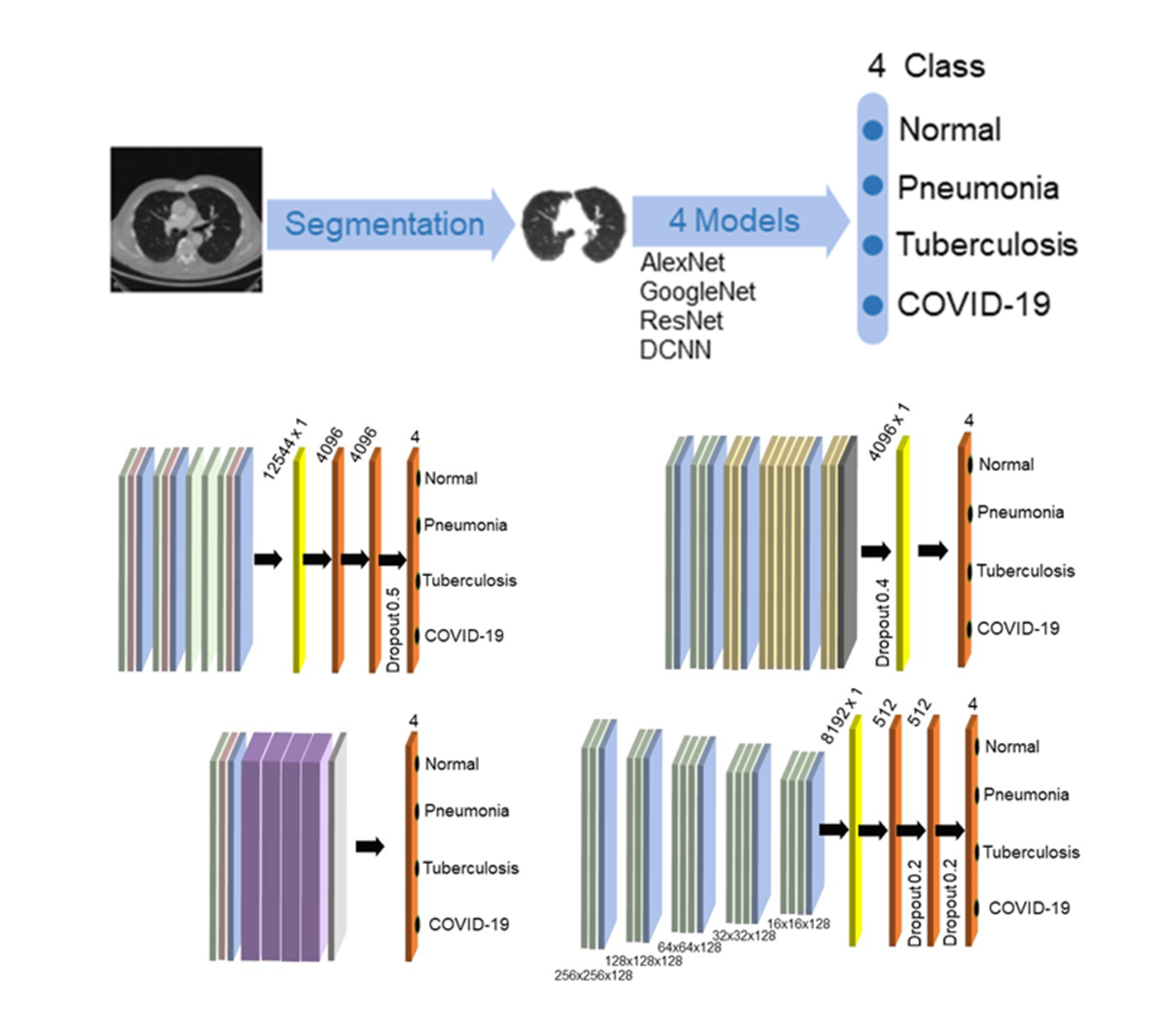
Downloads
Published
How to Cite
Issue
Section
License
Copyright (c) 2023 Walailak University

This work is licensed under a Creative Commons Attribution-NonCommercial-NoDerivatives 4.0 International License.