Rice Growth and Yield Responses to Climate Variabilities and Scenarios
DOI:
https://doi.org/10.48048/tis.2023.6390Keywords:
Biomass, Climate change, Crop modeling, CO2, Rice, Temperature, YieldAbstract
Rice is the essential food crop of An Giang Province. Vietnam and the whole world are facing several problems hindering climate change, such as increased temperature and CO2 concentration that many manufacturers’ companies and managers need to estimate output to make production plans or adjust policies. In this study, the model known as SIMPLE was applied to simulate the biomass and yield of rice in 2 crop seasons Autumn - Winter 2020 (AW) and Winter - Spring 2020 - 2021 (WS), in Cho Moi district, An Giang province, Vietnam (10° 23' 47"N, 105° 27' 41"E) and analyzed the effects of climate variabilities and scenarios on simulation results. Heat stress showed a relatively negative impact on the growth and development of rice in AW more seriously than WS due to climate variabilities. Climate change scenario RCP8.5 (RCP - Representative Concentration Pathway) has predicted that atmosphere temperature may increase above 4 °C and CO2 concentration to reach 900 ppm by the end of the 21st century. As a result, from the model, for every 100 ppm CO2 concentration increase, the cumulative rice biomass increased by 8 and 10 % in AW and WS, respectively. Moreover, conditions assumed from the model that increased 5 °C caused a decrease in cumulative biomass up to 7.2 % in AW season compared to 3.1 % in WS season. However, with responses of 5 °C increasing in the model, rice yield decreased relatively rapidly from 8.5 % in AW and 7 % WS.
HIGHLIGHTS
- The model known as SIMPLE has been used in this study
- RMSE of our model differs from the observed yield from 4.2 % (Winter-Spring crop-WS) to 5.5 % (Autumn-Winter crop-AW)
- For every 100-ppm CO2 concentration increased, the cumulative rice biomass increased by 8 and 10 % in AW and WS, respectively
- Increasing 5 °C, rice yield decreased 8.5 % in AW and 7 % WS
- Sensitivity analysis showed that RUE (Radiation Use Efficiency) has the most influencing factor on rice yield
GRAPHICAL ABSTRACT
Downloads
Metrics
References
General Statistics Office of Vietnam. Statistical yearbook of Vietnam. General Statistics Office of Vietnam, Ha Noi, Vietnam, 2021.
NV Manh, NV Dung, NN Hung, K Matti, M Bruno and A Heiko. Future sediment dynamics in the Mekong delta floodplains: Impacts of hydropower development, climate change and sea level rise. Global Planet. Change 2015; 127, 22-33.
D Eckstein, V Künzel and L Schäfer. Global climate risk index 2018. Germanwatch, Bonn, Germany, 2017.
S Chumkiew, M Jaroensutasinee and K Jaroensutasinee. Impact of global warming on coral reefs. Walailak J. Sci. Tech. 2011; 8, 111-29.
Nguyen, TH Nguyen, T Tran, TL Nguyen and VT Va. Climate change and impacts in Vietnam. Science and Technology, Vietnam, 2011.
W Cheng, H Sakai, K Yagi and T Hasegawa. Interactions of elevated (CO2) and night temperature on rice growth and yield. Agr. Forest Meteorol. 2009; 149, 51-8.
RA Betts, CD Jones, JR Knight, RF Keeling and JJ Kennedy. El Niño and a record CO2 rise. Nat. Clim. Change 2016; 6, 806-10.
W Wang, C Cai, J He, J Gu, G Zhu, W Zhang, J Zhu and G Liu. Yield, dry matter distribution and photosynthetic characteristics of rice under elevated CO2 and increased temperature conditions. Field Crops Res. 2020; 248, 107605.
CB Chisanga, M Moombe and E Phiri. Modelling climate change impacts on maize. CABI Rev. 2022; 17, 008.
F Ewert, MDA Rounsevell, I Reginster, MJ Metzger and R Leemans. Future scenarios of European agricultural land use: I estimating changes in crop productivity. Agr. Ecosyst. Environ. 2005; 107, 101-16.
G Hoogenboom, CH Porter, KJ Boote, V Shelia, PW Wilkens, U Singh, JW White, S Asseng, JI Lizaso and LP Moreno. The DSSAT crop modeling ecosystem. In: K Boote (Ed.). Advances in crop modelling for a sustainable agriculture. Burleigh Dodds Science Publishing, Cambridge, 2019, p. 173-216.
RC Izaurralde, WB McGill, JR Williams, CD Jones, RP Link, DH Manowitz, DE Schwab, X Zhang, GP Robertson and N Millar. Simulating microbial denitrification with EPIC: Model description and evaluation. Ecol. Model. 2017; 359, 349-62.
T Foster, N Brozović, AP Butler, CMU Neale, D Raes, P Steduto, E Fereres and TC Hsiao. Aqua Crop-OS: An open source version of FAO's crop water productivity model. Agr. Water Manag. 2017; 181, 18-22.
C Zhao, B Liu, L Xiao, G Hoogenboom, KJ Boote, BT Kassie, W Pavan, V Shelia, KS Kim, I Hernandez-Ochoa, D Wallache, HP Cheryl, COY Stocklef and S Asseng. A SIMPLE crop model. Eur. J. Agron. 2019; 104, 97-106.
JL Monteith. Light distribution and photosynthesis in field crops. Ann. Bot. 1965; 29, 17-37.
M Ahmed and S Ahmad. Systems modeling. Springer Nature, Singapore, 2020, p. 1-44.
J Ross. The radiation regime and architecture of plant stands. Springer, Dordrecht, Netherlands, 1981.
S Asseng, IAN Foster and NC Turner. The impact of temperature variability on wheat yields. Global Change Biol. 2011; 17, 997-1012.
M Bindi, L Fibbi, B Gozzini, S Orlandini and F Miglietta. Modelling the impact of future climate scenarios on yield and yield variability of grapevine. Clim. Res. 1996; 7, 213-24.
F Ewert, D Rodriguez, P Jamieson, MA Semenov, RAC Mitchell, J Goudriaan, JR Porter, BA Kimball, PJ Pinter, R Manderscheid, HJ Weigel, A Fangmeier, E Fereres and F Villalobos. Effects of elevated CO2 and drought on wheat: Testing crop simulation models for different experimental and climatic conditions. Agr, Ecosyst. Environ. 2002; 93, 249-66.
CHB Priestley and RJ Taylor. On the assessment of surface heat flux and evaporation using large-scale parameters. Mon. Weather Rev. 1972; 100, 81-92.
J Ritchie, D Godwin and S Otter-Nacke. CERES-wheat: A user-oriented wheat yield model. Preliminary Documentation. Michigan State University, Michigan, 1985.
MKV Ittersum, SM Howden and S Asseng. Sensitivity of productivity and deep drainage of wheat cropping systems in a Mediterranean environment to changes in CO2, temperature and precipitation. Agr. Ecosyst. Environ. 2003; 97, 255-73.
P Woli, JW Jones, KT Ingram and CW Fraisse, Agricultural reference index for drought (ARID). Agron. J. 2012; 104, 287-300.
CHB Priestley and RJF Taylor. On the assessment of surface heat flux and evaporation using large-scale parameters. Mon. Weather Rev. 1972; 100, 81-92.
P Steduto, TC Hsiao, D Raes and E Fereres. AquaCrop - The FAO crop model to simulate yield response to water: I. Concepts and underlying principles. Agron. J. 2009; 101, 426-37.
A Wnuk, A Górny, J Bocianowski and M Kozak. Visualizing harvest index in crops. Commun. Biometry Crop Sci. 2013; 8, 48-59.
BA Rani and N Maragatham. Effect of elevated temperature on rice phenology and yield. Indian J. Sci. Tech. 2013; 6, 5095-7.
MD Morris. Factorial sampling plans for preliminary computational experiments. Technometrics 1991; 33, 161-74.
C Xu and G Gertner. Understanding and comparisons of different sampling approaches for the fourier amplitudes sensitivity test (FAST). Comput. Stat. Data Anal. 2011; 55, 184-98.
VN Trụ and NTL Tuyết. Application of MORRIS method for analysis of sensitivity of the factors influencing flooding flows calculated from small basin. J. Constr. Sci. Tech. 2017; 11, 26-32.
Y Uno, SO Prasher, R Lacroix, PK Goel, Y Karimi, A Viau and RM Patel. Artificial neural networks to predict corn yield from compact airborne spectrographic imager data. Comput. Electron. Agr. 2005; 47, 149-61.
RH McCuen, Z Knight and AG Cutter. Evaluation of the Nash-Sutcliffe efficiency index. J. Hydrolog. Eng. 2006; 11, 597-602.
C Folberth, A Baklanov, J Balkovič, R Skalský, N Khabarov and M Obersteiner. Spatio-temporal downscaling of gridded crop model yield estimates based on machine learning. Agr. Forest Meteorol. 2019; 264, 1-15.
AVM Ines and JW Hansen. Bias correction of daily GCM rainfall for crop simulation studies. Agr. Forest Meteorol. 2006; 138, 44-53.
C Gornott and F Wechsung. Statistical regression models for assessing climate impacts on crop yields: A validation study for winter wheat and silage maize in Germany. Agr. Forest Meteorol. 2016; 217, 89-100.
FK Musyoka, P Strauss, G Zhao, R Srinivasan and A Klik. Multi-step calibration approach for SWAT model using soil moisture and crop yields in a small agricultural catchment. Water 2021; 13, 2238.
B Liu, S Asseng, C Müller, F Ewert, J Elliott, DB Lobell, P Martre, AC Ruane, D Wallach and JW Jones. Similar estimates of temperature impacts on global wheat yield by three independent methods. Nat. Clim. Chang 2016; 6, 1130-6.
R Confalonieri, G Bellocchi, S Tarantola, M Acutis, M Donatelli and G Genovese. Sensitivity analysis of the rice model WARM in Europe: Exploring the effects of different locations, climates and methods of analysis on model sensitivity to crop parameters. Environ. Model. Software 2010; 25, 479-88.
L Paleari and R Confalonieri. Sensitivity analysis of a sensitivity analysis: We are likely overlooking the impact of distributional assumptions. Ecol. Model. 2016; 340, 57-63.
X Hao, J Gao, X Han, Z Ma, A Merchant, H Ju, P Li, W Yang, Z Gao and E Lin, Effects of open-air elevated atmospheric CO2 concentration on yield quality of soybean (Glycine max (L.) Merr). Agr. Ecosyst. Environ. 2014; 192, 80-4.
MJ Morrison and DW Stewart, heat stress during flowering in summer Brassica. Crop Sci. 2002; 42, 797-803.
T Tran, VT Nguyen, TLH Huynh, VK Mai, XH Nguyen and HP Doan. Climate change and sea level rise scenarios for Vietnam. Ministry of Natural resources and Environment, Hanoi, Vietnam, 2016.
Z Jiang, SV Raghavan, J Hur, Y Sun, SY Liong, VQ Nguyen and TVP Dang. Future changes in rice yields over the Mekong River Delta due to climate change - alarming or alerting? Theor. Appl. Climatol. 2019; 137, 545-55.
T Yuliawan and I Handoko. The effect of temperature rise to rice crop yield in Indonesia uses Shierary Rice model with geographical information system (GIS) feature. Proc. Environ. Sci. 2016; 33, 214-20.
P Krishnan, DK Swain, BC Bhaskar, SK Nayak and RN Dash. Impact of elevated CO2 and temperature on rice yield and methods of adaptation as evaluated by crop simulation studies. Agr. Ecosyst. Environ. 2007; 122, 233-242.
J Xu, A Henry and N Sreenivasulu. Rice yield formation under high day and night temperatures - A prerequisite to ensure future food security. Plant, Cell Environ. 2020; 43, 1595-608.
W Wang, C Cai, SK Lam, G Liu and J Zhu. Elevated CO2 cannot compensate for japonica grain yield losses under increasing air temperature because of the decrease in spikelet density. Eur. J. Agron. 2018; 99, 21-9.
T Li, T Hasegawa, X Yin, Y Zhu, K Boote, M Adam, S Bregaglio, S Buis, R Confalonieri, T Fumoto, D Gaydon, M Marcaida III, H Nakagawa, P Oriol, AC Ruane, F Ruget, B Singh, U Singh, L Tang, F Tao, P Wilkens, H Yoshida, Z Zhang and B Bouman. Uncertainties in predicting rice yield by current crop models under a wide range of climatic conditions. Glob. Chang Biol. 2015; 21, 1328-41.
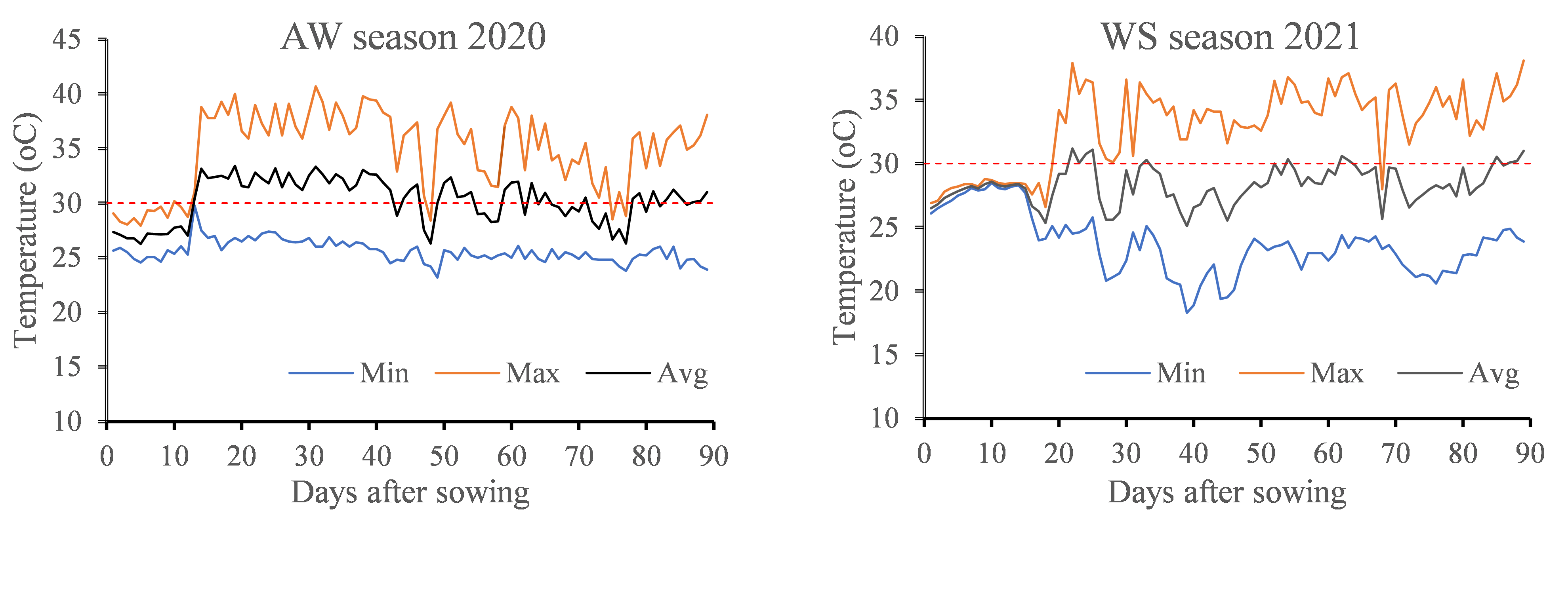
Downloads
Published
How to Cite
Issue
Section
License
Copyright (c) 2022 Walailak University

This work is licensed under a Creative Commons Attribution-NonCommercial-NoDerivatives 4.0 International License.