Spline Model in The Case of Cervical Cancer Patient Resilience
DOI:
https://doi.org/10.48048/tis.2023.6170Keywords:
Cancer, Cervical, Cox, Model, Spline, Survival, WomenAbstract
Cervical cancer ranks highest in developing countries, and ranks 10th in developed countries as a cause of death for women. In Indonesia, cervical cancer ranks second out of the 10 most common cancers based on Anatomical Pathology data in 2010 with an incidence rate of 20 %. The number of new women with cervical cancer ranges from 90 - 100 cases per 100,000 population and every year there are 40 thousand cases of cervical cancer. Cervical cancer is a gynecological disease that has a high level of malignancy and is caused by the Human Papilloma Virus (HPV). One method that can be used in the analysis of human survival is survival analysis using the Cox proportional hazard model. This research will use the development of the Cox model using the Spline function as its basis. Based on the results obtained, the developed model can model the data well and give a low MSE. Based on the developed model, it is found that the variables that influence the survival rate of cervical cancer patients are age, stage, type of PRC transfusion treatment and comorbidities.
HIGHLIGHTS
- Due to in silico study such as molecular docking, molecular dynamic simulation is an important computational method for drug design. It can be used to predict the binding orientation of small molecule drug candidates to their protein targets. In addition, docking is also can be applied to predict small molecule affinity and activity. Thus, molecular docking has an important role in drug discovery and rational design
- The ligand interaction with the receptor was more stable due to the decreased binding free energy value
- The lowest binding free energy and lowest RMSD value of this molecule, which may presumably cause compound 3 to has better binding affinity compare to other compounds
GRAPHICAL ABSTRACT
Downloads
Metrics
References
K Dauda, WB Yahya and A Banjoko. Survival analysis with multivariate adaptive regression using cox-snell residual. Anale Seria Informatică 2015; 13, 25-41.
G Yaprak, D Tataroglu, B Dogan and M Pekyurek. Prognostic factors for survival in patients with gastric cancer: Single-centre experience. N. Clin. Istanbul 2020; 7, 146-52.
J Faradmal, A Talebi, A Rezaianzadeh and H Mahjub. Survival analysis of breast cancer patients using cox and frailty models. J. Res. Health Sci. 2012; 12, 127-30.
MA Pourhoseingholi, E Hajizadeh, DB Moghimi, A Safaee, A Abadi and MR Zali. Comparing cox regression and parametric models for survival of patients with gastric carcinoma. Asian Pac. J. Canc. Prev. 2007; 8, 412-6.
B Moghimi-Dehkordi, A Safaee, MA Pourhoseingholi, R Fatemi, Z Tabeie and MR Zali. Statistical comparison of survival models for analysis of cancer data. Asian Pac. J. Canc. Prev. 2008; 9, 417-20.
A Perperoglou, A Keramopoullos and HC Houwelingen. Approaches in modelling long-term survival: An application to breast cancer. Stat. Med. 2007; 26, 2666-85.
I Pelagia. 2016, Variable selection of fixed effects and frailties for cox proportional hazard frailty models and competing risks frailty models. Ph. D. Dissertation. University of Manchester, Manchester, England.
P Naseri, AR Baghestani, N Momenyan and ME Akbari. Application of a mixture cure fraction model based on the generalized modified weibull distribution for analyzing survival of patients with breast cancer. Int. J. Canc. Manag. 2018; 11, e62863.
A Kavkler, D Daniela-Emanuela, AG Babucea, I Bicanic, D Tevdovski, K Tosevska-Trpcevska and D Boršič. Cox regression models for unemployment duration in Romania, Austria, Slovenia, Croatia, and Macedonia. Rom. J. Econ. Forecast. 2009; 10, 81-104.
SM Grybach, LZ Polishchuk and VF Chekhun. Analysis of the survival of patients with breast cancer depending on age, molecular subtype of tumor and metabolic syndrome. Exp. Oncol. 2018; 40, 243-8.
SC Robles and E Galanis. Breast cancer in latin america and the caribbean. Revista Panamericana Salud Publica 2002; 11, 178-85.
RO Ferraz and DC Moreira-Filho. Survival analysis of women with breast cancer: Competitive risk models. Cien Saude Colet 2017; 22, 3743-54.
M Liu, L Li, W Yu, J Chen, W Xiong, S Chen and L Yu. Marriage is a dependent risk factor for mortality of colon adenocarcinoma without a time-varying effect. Oncotarget 2017; 8, 20056-66.
JY Charati, G Janbabaei, N Alipour, S Mohammadi, SG Gholiabad and A Fendereski. Survival prediction of gastric cancer patients by artificial neural network model. Gastroenterol. Hepatol. Bed Bench 2018; 11,110-7.
R Kelter. Bayesian survival analysis in STAN for improved measuring of uncertainty in parameter estimates. Measurement: Inter. Res. and Persp. 2020; 18, 101-9.
J Lu, L Cao, CH Zheng, P Li, JW Xie, JB Wang, JX Lin, QY Chen, M Lin and RH Tu. The preoperative frailty versus inflammation-based prognostic score: Which is better as an objective predictor for gastric cancer patients 80 years and older? Ann. Surg. Oncol. 2017; 24, 754-62.
Y Fujisawa, S Yoshikawa, A Minagawa, T Takenouchi, K Yokota, H Uchi, N Noma, Y Nakamura, J Asai, J Kato, S Fujiwara. Clinical and histopathological characteristics and survival analysis of 4594 Japanese patients with melanoma. Cancer med. 2019; 8, 2146-56.
DR Arifanti and R Hidayat. Statistical inference on nonparametric spline models and its applications. J. Phys. Conf. 2021; 2123, 012023.
JD Opsomer and D Ruppert. Fitting a bivariate additive model by local polynomial regression. Ann. Stat. 1997; 25, 186-211.
JM Maronge, Y Zhai, DP Wiens and Z Fang. Optimal designs for spline wavelet regression models. J. Stat. Plann. Infer. 2017; 184, 94-104.
A Antoniadis, J Bigot and T Sapatinas. Wavelet estimators in nonparametric regression: A comparative simulation study. J. Stat. Software 2001; 6, 1-83.
A Antoniadis and F Leblanc. Nonparametric wavelet regression for binary response. Statistics 2000; 34, 183-213.
R Hidayat, Ma’rufi and Yuliani. Integral estimator with kernel approach for estimating nonparametric regression functions. J. Phys. Conf. 2021; 2123, 012022.
IN Budiantara, V Ratnasari, M Ratna and I Zain. The combination of spline and kernel estimator for nonparametric regression and its properties. Appl. Math. Sci. 2015; 9, 6083-94.
V Ratnasari, IN Budiantara, M Ratna and I Zain. Estimation of nonparametric regression curve using mixed estimator of multivariable truncated spline and multivariable kernel. Global J. Pure Appl. Math. 2016; 12, 5047-57.
R Hidayat, IN Budiantara, BW Otok and V Ratnasari. The regression curve estimation by using mixed smoothing spline and kernel (MsS-K) model. Comm. Stat. Theor. Meth. 2021; 50, 3942-53.
IN Budiantara, V Ratnasari, M Ratna, W Wibowo, N Afifah, DP Rahmawati, MAD Octavanny. Modeling percentage of poor people in indonesia using kernel and fourier series mixed estimator in nonparametric regression. Invest. Operacional. 2019; 40, 538-50.
H Nurcahayani, IN Budiantara and I Zain. The curve estimation of combined truncated spline and fourier series estimators for multi-response nonparametric regression. Mathematics 2021; 9, 1141.
R Hidayat, IN Budiantara, BW Otok and V Ratnasari. An extended model of penalized spline with the addition of kernel functions in nonparametric regression model. Appl. Math. Inform. Sci. 2019; 13, 18.
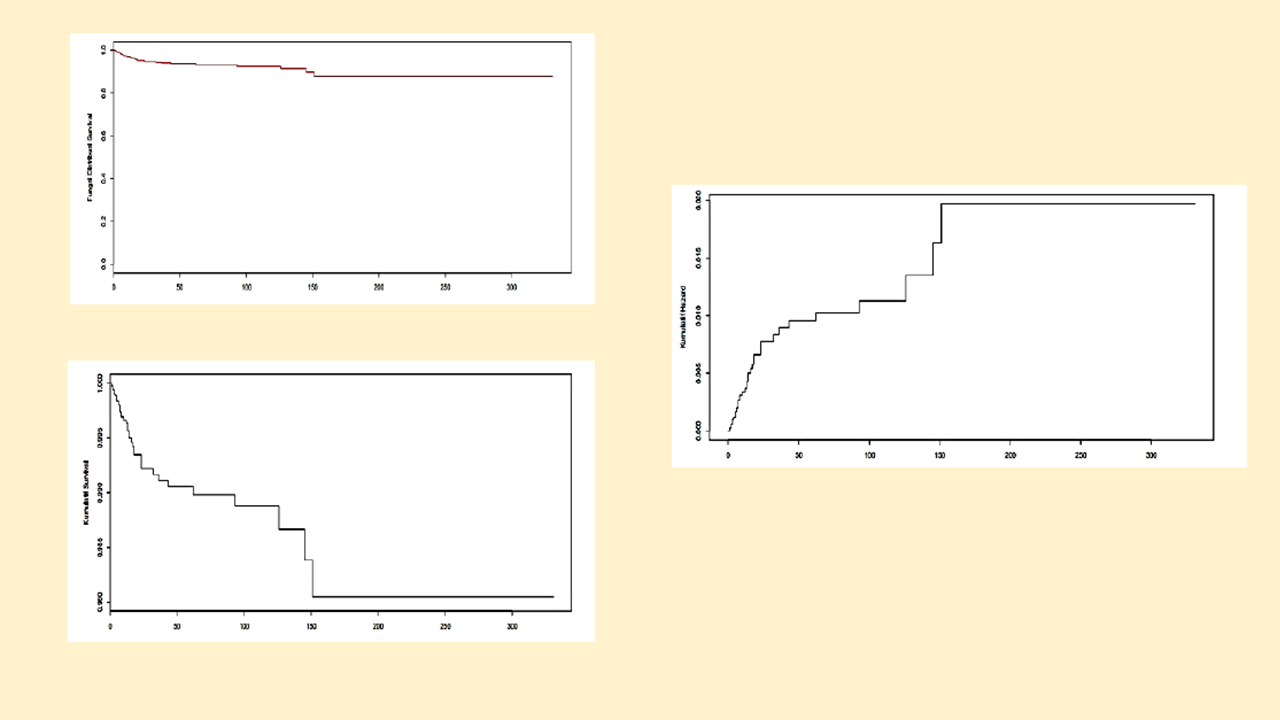
Downloads
Published
How to Cite
Issue
Section
License
Copyright (c) 2023 Walailak University

This work is licensed under a Creative Commons Attribution-NonCommercial-NoDerivatives 4.0 International License.